Abstract
The use of artificial intelligence in different parts of human life is becoming inevitable and it is expected that in the near future, the range of artificial intelligence applications will include all service, industrial, research, and educational activities. Providing a solution or performance enhancement in research and industrial activities, considering that these activities contain lots of dependent parameters with formulated/non-formulated correlations, is always a challenge for researchers. The recent approach of researchers in using statistical data and applying techniques based on artificial intelligence is a promising solution that provides the desired answers more quickly and accurately. The automobile industry and internal combustion engines have also benefited from the advantages of artificial intelligence in order to improve their performance and efficiency. Among the most important developments and achievements of this approach, we can mention real-time modeling, optimization and intelligent control, new fuel combinations, fault detection systems, and self-driving vehicles. Therefore, in this chapter, the recent research and industrial achievements of diesel engines due to the use of artificial intelligence techniques will be discussed.
Keywords
- artificial intelligence
- machine learning
- deep learning
- diesel engine
- automotive industry
1. Introduction
Nowadays, the applications of Artificial Intelligence (AI) are noticeably extending in the wide range of research and industries. It seems that industrial products are competing in equipping themselves with artificial intelligence. Diesel engines also use this concept to improve their performance, usability, and viability in this competition. Consequently, in this chapter, the development of diesel engines via artificial intelligence will be discussed. First, the fundamentals of artificial intelligence, classifications, and applications are briefly illustrated, then a short review about employing artificial intelligence in internal combustion engines is presented, and finally, the recent trends, achievements, and challenges of using artificial intelligence in diesel engines are discussed.
2. Artificial intelligence
Artificial intelligence is considered as an effort to persuade a computer, robot, or other piece of technology to think and process data just similar to human’s brain. So, artificial intelligence has to find how the human brain thinks, learns, and makes decisions during processes like solving a problem or executing a task. The aim of AI is to develop products by adding functionality related to human acts of reasoning, learning, and problem-solving [1].
2.1 Types of artificial intelligence
While artificial intelligence can be divided into various types, there are two main classifications which are based on its capability and functionally and are shown in Figure 1. Based on the capabilities, it is divided into three types namely; Narrow, General, and Super AI. The most common and available type of AI is Narrow type which is able to perform a dedicated task with intelligence. It is only trained for a specific task and could fail in unpredictable ways if it goes beyond its limits. Apple Siriis, self-driving cars, and speech/image recognition are the most well-known examples of Narrow AI. General AI could perform intellectual tasks with efficiency like a human and Super AI is an upper-level of human Intelligence. It is able to perform any task better than humans with cognitive properties. Up to now, there is no executed example of General and Super AIs and researchers are working on the General level of AI [2].
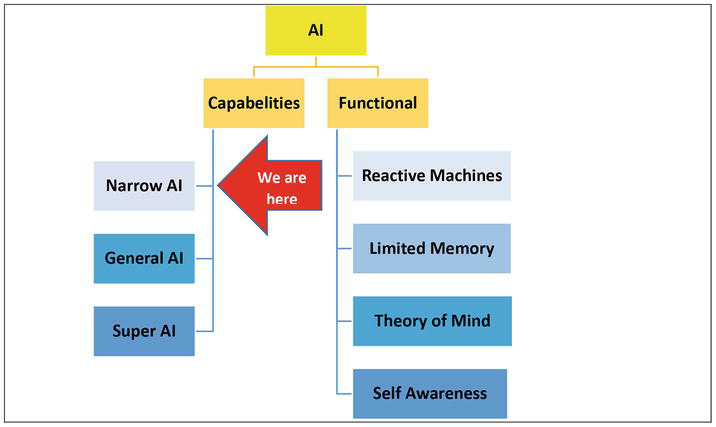
Figure 1.
Classifications of artificial intelligence.
The other classifications of AI systems are based on their function. In this approach, it is divided into four classes namely; Reactive Machines, Limited Memory, Theory of Mind, and Self Awareness. The simplest AI in this approach is the Reactive machine which reacts based on the current scenario and best possible action. It does not use previous experiences to make decisions. Limited Memory AI could store and use previous experiences and or some data for a limited short period of time to make decisions. The most famous example is self-driving cars which store the recent speed of nearby cars, the distance of other cars, speed limit, and other information to navigate the road. Theory of Mind AI is able to understand human emotions and beliefs like humans, and Self Awareness AI which is known as the future of artificial intelligence and will be smarter than the human mind. Both of them do not exist in reality and researchers are working on them [2].
2.2 AI vs. machine learning
One of the common mistakes in artificial intelligence subject is using AI, Machine Learning (ML), and Deep Learning interchangeably. These terms are actually distinct but related concepts and have meaningful differences. Simply, AI could be considered as computer software that imitates humans thinking, analyzing, reasoning, and learning processes in order to handle complex duties. Machine learning as the subset of AI is actually the model producer using special learning algorithms based on data which makes AI able to perform such complex tasks. Up to now, most applied AIs are provided using machine learning, so it is the main reason for using them synonymously. However, AI refers to the comprehensive concept of making human-like perceptions by computer software, while machine learning refers to only one method or model of doing that. Figure 2 indicates the relations between Al and ML [3].

Figure 2.
AI vs. ML.
2.3 The applications of AI
The usage of products and services that are using AI is such a common that one may not be aware of engaging with AI during his daily life. In fact, AI-powered products and services have been increasingly intertwined with human daily life. Banking applications that checks shady transactions, spam detectors that keeps our inbox free of virus, and video-streaming platforms which recommend us to watch an attractive movie are just some examples of our daily routines which are equipped with AI. There is no doubt that one of the most valuable achievements of AI is the development of services in health care. ML models capable of analyzing the medical pictures for cancerous growths, smartphone applications which provide personalized treatment schedules, and automation systems which efficiently allocate hospital resources are just some of the achievements in this subject. Furthermore, AI has had a significant impact on the world-class business. It has been used to reduce the costs using automation and to produce actionable insights via analyzing big data sets [4]. Some of the most applications of AI in our daily life are shown in Figure 3.
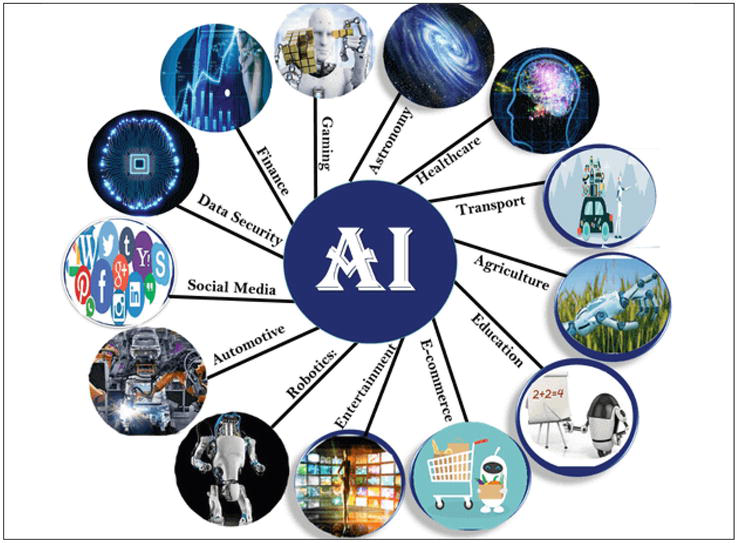
Figure 3.
Top applications of AI.
3. AI in internal combustion engines
It could be asserted that the world is experiencing the midst of a third wave of artificial intelligence algorithm development, after the first and second ones in the 1970s and 2000s [5]. Besides the conventional ways to improve engine performance, its numerous challenges and the competition between the producers lead the researchers to develop artificial intelligence algorithm and apply them in the engine industry. Using conventional methods to regulate, anticipate, and optimize the hardly nonlinear and complex events of an ICE such as 2D and 3D mapping of the engine characteristics, large number of nonlinear combustion chemical reactions, pressure and temperature gradients, multi-phase flow interactions, and formation of particulate matters is a challenging issue. So, the models based on AI could estimate the engine performance ignoring such hard phenomena and just based on the experimental data.
Furthermore, there are some ignored issues in conventional ICE management systems such as regulating stochastic cyclic variability that brings poor performance for the engine management systems. More powerful and efficient control models are provided using artificial intelligent algorithms in various ICE combustion modes such as homogeneous charge compression ignition or reactivity-regulated compression ignition. The rapid rise of employing and applying big data, make us able to provide ever-more sufficient and smart management system using a large amount of details and countless information from the engine such as speed, load, indicated torque, temperature, pressure, fuel injection, fuel consumption, etc. It is obvious that such an enormous amount of data needs a powerful analyzing tool and artificial intelligence algorithms are the best choice considering efficiency, time consumption, errors, and cost for engine management. In addition, AI technology using the data adopted from noticeable comparable ICEs connected to a network could provide a real-time smart engine management system.
3.1 Recent trend
The ever-increasing growth of human societies, continuous change of customer desirers and the mechanization of traditional common methods besides fossil fuel resource limitations, environmental pollutants concerns, and design considerations lead researchers to have non-stop efforts developing the performance of internal combustion engines. Extended works have been carried out by researchers to have more efficient and cleaner engines [6, 7, 8]. These efforts contain a vast variety of approaches from engine design [9, 10] to fuel development [11, 12] and combustion control [13, 14].
Among these extended subjects, applying AI to internal combustion engines is a new approach on which researchers are working [15, 16]. Although the most of efforts have been devoted to the control approaches [17, 18], the range of employing AI in engine performance development is still wide from the optimized redesign [19, 20] to fuel/charge mixture [21, 22] and combustion characteristics [23, 24]. Furthermore, due to the strong research background in this field, it can be asserted that AI technology is simultaneously applied to all known combustion modes, from spark ignition engines [25, 26] to diesel [27, 28] and low-temperature combustion ones [29, 30]. Most of the efforts are based on the Artificial Neural Network (ANN) to simulate the engine performance and then use such a model for control approaches or optimization. Indeed, engine general performance such as power, torque, and emission based on its operating conditions and input parameters such as valve timing, ambient pressure/temperature, and equivalence ratio could be estimated using machine learning and deep learning techniques. In such models, based on the engine data set, some predictive correlations will be produced and used to estimate the target values based on the input ones. For example in Ref. [30], the start of combustion has been estimated based on the ambient pressure, ambient temperature, inlet valve closing, equivalence ratio, engine speed, and compression ratio via a simple linear correlation suggested by ML regression models for methane fueled homogeneous charge compression ignition engines. Such a correlation is useful to control the engine combustion stability in the real-time application. Or, in Ref. [29] the combustion noise level of a converted-diesel homogeneous charge compression ignition engine is estimated by the ANN model using engine experimental data. This real-time predictor is able to predict the noise level with 5% accuracy, so applying it to the engine control strategy may protect the engine from intense ringing. Although a huge number of works in this field are presented in the literature, generally they can be categorized into four section namely; performance simulation, control approaches, fault diagnosis, and optimization as shown in Figure 4.

Figure 4.
AI technology in internal combustion engines.
4. AI in diesel engines
Having more intelligent vehicles supervised, unsupervised, and reinforcement learning techniques are employed by machine learning algorithms and deep learning models. Both supervised and unsupervised learning techniques, such as regression, classification, detection, segmentation, dimensionality reduction, and clustering use the data obtained from various sensors located in different parts of engines to analyze the factors affecting fuel consumption, emissions, fault detection, maintenance, understanding the driver’s behavior, automated driver assistance systems, and self-driving. For autonomous vehicles, considering the decision-making process based on the environmental condition, path planning, object detection, and decision-making control, it is more common to use semi-supervised learning, reinforcement learning, and deep learning techniques. The domain of efforts employing these artificial intelligence techniques on diesel engines is categorized into five classes namely; fuel construction, engine performance, fault diagnosis, engine control, and optimization which are illustrated here. It should be noted that the research at this level can be considered in primary steps since just some AI techniques have been employed to develop diesel engines as shown in Figure 5.

Figure 5.
AI in diesel engines.
4.1 Fuel construction
Fossil fuels play an important role in providing the demanded energy for almost all sectors of life after the globalization of the world and the industrial revolution. However, there are extended efforts to find other sources of energy due to their detrimental impact on the global ecosystem. For the transportation sector, biodiesel as a promising replacement for fossil fuel-based diesel is becoming more popular as its performance is known more. As the recent achievements, a huge number of techniques have been provided to efficiently extract biodiesel from different oils/fats. Using AI approaches, we are able to estimate the efficiency of biodiesel production techniques, optimize such a process, and minimize process costs. The AI-powered biodiesel performance prediction models have several stages namely; data collection, data preprocessing, developing, applying ML algorithm on biodiesel data, and finally predicting the target values such as unknown biodiesel characteristics and properties. Consequently, the main achievements of using AI in the biodiesel production process could be reported as the improvement of the process optimization, development of the fuel properties predictor models, and ultimately cost reduction [31].
4.2 Engine performance
It can be asserted that one of the most focused sections of AI applications in diesel engines is the engine performance estimation using the engine simulator models. Based on the target of research, the engine outputs such as fuel consumption, exhaust emission, brake torque, and power could be estimated using AI techniques due to the engine inlet parameters. Although most of this research is carried out by the ANN, there are some other efforts employing different methods such as Adaptive-Neuro Fuzzy Inference System (ANFIS) [32] and Gene Expression Programming (GEP) [33, 34] to compare the accuracy and comprehensibility of AI techniques on the performance prediction of diesel engines.
4.2.1 Fuel consumption
Fuel consumption, as a key factor of vehicles especially for heavy-duty ones, directly affects on overall operational cost of vehicles. The fuel economy standards of vehicles are regulated by the Corporate Average Fuel Economy (CAFE) standards of the National Highway Traffic Safety Administration (NHTSA) of the United States. It is obvious that noticeable savings in the transportation industry are achievable by improving fuel efficiency and reducing fuel consumption, so applying AI techniques could create a huge revolution on this issue. Several research and studies have been carried out to model the fuel consumption/fuel efficiency of diesel engines based on statistical and other AI approaches. Furthermore, estimating the fuel efficiency could help the transportation fleet management and for diagnostic targets wherever we are facing with high level of fuel consumption. While the statistical and physics-based approaches are time-consuming, AI and machine learning methods have high speed and are more accurate for such modeling. In consequence, several studies have been performed to predict fuel consumption in vehicles using machine learning and deep learning techniques such as Support Vector Machine (SVM) [35], Random Forest (RF) [36], and ANN [37, 38, 39].
4.2.2 Exhaust emission
Emission reduction has been significantly focused on by the automotive industries since the emission regulations for transportation sectors were published. The standards of environmental protection and emission inventories could be developed by emission efficiency estimation. Considering the challenges of physics-based models, the research and development section of companies prefer to use data-driven approaches to estimate emissions and in the next step to reduce the emissions. Previous research for analysis and estimation emissions in diesel engines such as carbon monoxide, carbon dioxide, nitrogen oxides, hydrocarbon, and particulate matter based on machine learning and AI techniques have proved the power of AI in emissions data investigations [40, 41, 42]. These studies have motivated the use of artificial intelligence techniques such as ANN, ANFIS, SVM, RF, and Deep Neural Networks (DNN) [43] in analyzing and estimating of emissions for diesel engines.
4.3 Fault diagnosis
Any computerized or even manual evaluation to detect potential issues leading to any malfunction such as misfire or knock is defined as engine diagnosis in the literature. Misfire is considered a common failure situation of diesel engines which could bring a significant reduction in the brake torque, power, and economic performance, so numerous research is devoted to this challenge. It could occur in diesel engines due to poor fuel quality, unfavorable fuel injection, and electro-mechanical failure. Indeed, misfires might cause irregular pollution and operation in diesel engines. Therefore, researchers are continuously working to provide real-time and accurate misfire detection models [44] using ANN [45] and RF [36] techniques. Even if modern diesel engines are equipped by semi-intelligent electronic control units that are using AI techniques based on the data driven from numerous sensors, misfire occurrences are still hard to identify using a simple algorithm or reasoning. Both of electrical or mechanical component failures such as injection nozzle blockage, solenoid drive failure, and unfavorable air-fuel mixing injection may cause misfires in diesel engines which are pretty hard to detect and manage.
Engine knock, as the other engine fault, is a tapping-pinging sound due to the unwanted auto-ignition of the air-fuel mixture that gets louder as the driver requests more acceleration by pushing the throttle pedal. The result of such an unpunctual combustion is a shock wave which causes the in-cylinder pressure to rise quickly and if a significant knock is not removed and managed soon, the engine will experience damage to rings, pistons, and exhaust valves. Consequently, extended research are devoted to knock detection-based AI techniques such as Convolutional Neural Network (CNN) [46] and RF learning [47]. Furthermore, there are some efforts to detect the intake system leakage [48] and oxygen [49] as the other faults of diesel engines are mainly based on ANN.
4.4 Engine control
Other approach to improve the performance of diesel engines is applying efficient controller and controlling the operating parameters. Having better control of automobiles and improving engine performance, the electronic systems could be integrated with vehicle operation and control. In this approach, the control process of the vehicle operation will be carried out automatically using several sensors and associated electronic transducer systems. One of the recent achievements in this field could be reported Diesel Particulate Filter (DPF) device which is designed to remove diesel PM or soot from the exhaust gases of diesel engines. In addition, an electronic diesel injection controller designed for precise metering and fuel delivery to the combustion chamber could be found in recent trucks and cars using diesel engines.
Despite extensive research [50, 51] carried out in this domain, it seems that more investigations are still needed in two main areas namely; modeling of engine performance under a wide range of operating conditions and development of an intelligent vehicle control system incorporating such a model to improve vehicle overall performance.
4.5 Optimization
The other applications of AI techniques include ANN, Fuzzy, Genetic Algorithm (GA), and Particle Swarm Optimization (PSO) in diesel engines is employing them to optimize the engine operating conditions to achieve the desired target values. Extended research has been carried out in this field, and researcher are continuously developing the optimization techniques as well as the engine performance. It can be asserted that optimization research is applied to almost all aspects of diesel engines and experiencing nonstop development. Indeed, it seems that intelligence optimization is entering into a new phase of developing the real-time optimization. In this approach, the target values may change during the time, thus the optimum values will be continuously updated. Researcher are not stopped at this point and are trying to apply such an approach to diesel engine calibration [52], but it should be noted that this is still of the primary steps and lots of effort are needed to have fully smart engine.
5. Conclusion
In this chapter, the fundamentals of artificial intelligence, the recent trends of using artificial intelligence in internal combustion engines, focused on diesel engines, achievements, and challenges have been discussed. To conclude the subject, it is better to look in more detail at the current technology and the basic definition of artificial intelligence.
As we are able to find in the literature, the current technology and research are focused on the modeling state and we are trying to apply the results to control approaches. As an example, when we introduce a semi-empirical correlation to estimate the out power of the engine based on AI/ML techniques, the main question is about intelligence! What is the difference between these correlations and other physics-based simulation results adopted from a 3-dimensional computational fluid dynamic or a quasi-dimensional thermodynamic model? If just the computational speed/limitations are the difference, where is the intelligence? Or when just the optimized condition is defined as a target, using AI-based optimization cannot mean the intelligence! The intelligence means the target may be updated due to the new condition or engine by the engine.
Looking at more details on the engine control unit, we are able to find some primary levels of intelligence. There are lots of calibration tables for operating ranges of the engine that the engine control unit uses to calculate the needed, for example, fuel to be injected based on the working condition and driver request. Based on the data adopted from the sensors (engine speed, manifold pressure and temperature, the angle of throttle, etc.) the coefficients of fuel mass flow rate correlation is found from the related calibration tables, and after applying the modification coefficients (liquid film loss) the demanded fuel mass flow rate to be injected and will be calculated. There are some other correlations and modifications based on the voltage of the battery to convert the demanded mass flow rate to the millisecond needed to open the injector. Finally, the feedback of such a process can be achieved using oxygen sensor by calculating the equivalence ratio. The schematic of the sensors and actuators for the fuel system is shown in Figure 6.
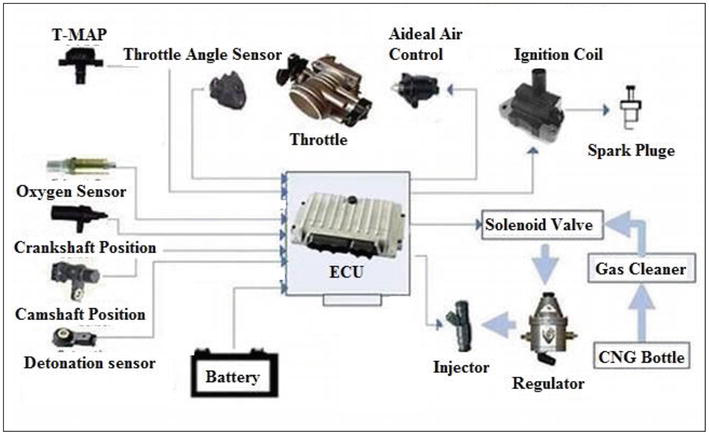
Figure 6.
The schematic of the fuel system.
This is an example of the primary level of artificial intelligence used in engines, the engine is able to find the needed inputs and apply them to achieve the target values. However, it is not the desired definition of a smart engine! The main issue is the process of these calculations which are mainly based on the calibration coefficients that had been defined during the experimental tests of engine in the test cell, and the engine configuration may change during the usage period or due to maintenance operations. So how the engine is able to detect such changes, and how it can find the new best target values, correlations, and their coefficients? In my idea, the right place of “intelligence” is exactly here. As the future development step, the engine control unit could be powered by AI to detect new conditions (engine by engine and even situation by situation), to define new best values of the targets (performance, emission, and faults), and to update the correlations for new values. In this case, we will be able to assert that we have a really smart engine!
References
- 1.
Chowdhary KR. Fundamentals of Artificial Intelligence. New Delhi: Springer India; 2020. pp. 603-649 - 2.
Müller, V.C. ed., 2016. Fundamental Issues of Artificial Intelligence (Vol. 376, p. 520). Berlin: Springer. - 3.
Khanzode KCA, Sarode RD. Advantages and disadvantages of artificial intelligence and machine learning: A literature review. International Journal of Library & Information Science (IJLIS). 2020; 9 (1):3 - 4.
Hall W, Pesenti J. Growing the Artificial Intelligence Industry in the UK. UK: Department for Digital, Culture, Media and Sport (United Kingdom); 2017 - 5.
Makridakis S. The forthcoming artificial intelligence (AI) revolution: Its impact on society and firms. Futures. 2017; 90 :46-60 - 6.
Khan MY, Giordano M, Gutierrez J, Welch WA, Asa-Awuku A, Miller JW, et al. Benefits of two mitigation strategies for container vessels: Cleaner engines and cleaner fuels. Environmental Science & Technology. 2012; 46 (9):5049-5056 - 7.
Namar MM, Jahanian O, Shafaghat R, Nikzadfar K. Numerical/experimental study on downsized iranian national engine (ef7) performance at low engine speeds. International Journal of Engineering. 2021; 34 (9):2137-2147 - 8.
Köten H, Parlakyiğit AS. Effects of the diesel engine parameters on the ignition delay. Fuel. 2018; 216 :23-28 - 9.
Namar MM, Jahanian O, Shafaghat R, Nikzadfar K. Engine downsizing; global approach to reduce emissions: A world-wide review. HighTech and Innovation Journal. 2021; 2 (4):384-399 - 10.
Aljaghtham M, Celik E. Design optimization of oil pan thermoelectric generator to recover waste heat from internal combustion engines. Energy. 2020; 200 :117547 - 11.
Namar MM, Jahanian O. Energy and exergy analysis of a hydrogen-fueled HCCI engine. Journal of Thermal Analysis and Calorimetry. 2019; 137 :205-215 - 12.
Koten H, Yilmaz M, Zafer Gul M. Compressed biogas-diesel dual-fuel engine optimization study for ultralow emission. Advances in Mechanical Engineering. 2014; 6 :571063 - 13.
Namar MM, Jahanian O. A simple algebraic model for predicting HCCI auto-ignition timing according to control oriented models requirements. Energy Conversion and Management. 2017; 154 :38-45 - 14.
Yilmaz M, Köten H, Gul MZ. Effects of the injection parameters and compression ratio on the emissions of a heavy-duty diesel engine. International Journal of Vehicle Design. 2012; 59 (2/3):147-163 - 15.
Ghenai C, Husein LA, Al Nahlawi M, Hamid AK, Bettayeb M. Recent trends of digital twin technologies in the energy sector: A comprehensive review. Sustainable Energy Technologies and Assessments. 2022; 54 :102837 - 16.
Badra J, Owoyele O, Pal P, Som S. A machine learning-genetic algorithm approach for rapid optimization of internal combustion engines. In: Artificial Intelligence and Data Driven Optimization of Internal Combustion Engines. Netherland: Elsevier; 2022. pp. 125-158 - 17.
Aliramezani M, Koch CR, Shahbakhti M. Modeling, diagnostics, optimization, and control of internal combustion engines via modern machine learning techniques: A review and future directions. Progress in Energy and Combustion Science. 2022; 88 :100967 - 18.
Maldonado B, Stefanopoulou A, Kaul B. Artificial-intelligence-based prediction and control of combustion instabilities in spark-ignition engines. In: Artificial Intelligence and Data Driven Optimization of Internal Combustion Engines. Netherland: Elsevier; 2022. pp. 185-212 - 19.
Özmen Mİ, Cihan Ö, Kutlar A, Özsoysal OA, Baykara C. Modelling a single-rotor Wankel engine performance with artificial neural network At middle speed range. International Journal of Automotive Science And Technology. 2020; 4 (3):155-163 - 20.
Schuchter F, Bause K, Albers A. Artificial intelligence in hybrid vehicle transmission control-literature review and research methodology. In: 2021 International Symposium on Computer Science and Intelligent Controls (ISCSIC). USA: IEEE; 2021. pp. 303-307 - 21.
Kuzhagaliyeva N, Horváth S, Williams J, Nicolle A, Sarathy SM. Artificial intelligence-driven design of fuel mixtures. Communications Chemistry. 2022; 5 (1):111 - 22.
Ghaderi M, Javadikia H, Naderloo L, Mostafaei M, Rabbani H. An analysis of noise pollution emitted by moving MF285 tractor using different mixtures of biodiesel, bioethanol and diesel through artificial intelligence. Journal of Low Frequency Noise, Vibration and Active Control. 2019; 38 (2):270-281 - 23.
Kalogirou SA. Artificial intelligence for the modeling and control of combustion processes: A review. Progress in Energy and Combustion Science. 2003; 29 (6):515-566 - 24.
Zheng Z, Lin X, Yang M, He Z, Bao E, Zhang H, et al. Progress in the application of machine learning in combustion studies. ES Energy & Environment. 2020; 9 (2):1-14 - 25.
Mehra RK, Duan H, Luo S, Rao A, Ma F. Experimental and artificial neural network (ANN) study of hydrogen enriched compressed natural gas (HCNG) engine under various ignition timings and excess air ratios. Applied Energy. 2018; 228 :736-754 - 26.
Liu Z, Zuo Q , Wu G, Li Y. An artificial neural network developed for predicting of performance and emissions of a spark ignition engine fueled with butanol–gasoline blends. Advances in Mechanical Engineering. 2018; 10 (1):1687814017748438 - 27.
Hoang AT, Nižetić S, Ong HC, Tarelko W, Le TH, Chau MQ , et al. A review on application of artificial neural network (ANN) for performance and emission characteristics of diesel engine fueled with biodiesel-based fuels. Sustainable Energy Technologies and Assessments. 2021; 47 :101416 - 28.
Ağbulut Ü, Gürel AE, Sarıdemir S. Experimental investigation and prediction of performance and emission responses of a CI engine fuelled with different metal-oxide based nanoparticles–diesel blends using different machine learning algorithms. Energy. 2021; 215 :119076 - 29.
Bahri B, Shahbakhti M, Aziz AA. Real-time modeling of ringing in HCCI engines using artificial neural networks. Energy. 2017; 125 :509-518 - 30.
Namar MM, Jahanian O, Koten H. The start of combustion prediction for methane-fueled HCCI engines: Traditional vs. machine learning methods. Mathematical Problems in Engineering. 2022; 2022 :1 - 31.
Ahmad J, Awais M, Rashid U, Ngamcharussrivichai C, Naqvi SR, Ali I. A systematic and critical review on effective utilization of artificial intelligence for bio-diesel production techniques. Fuel. 2023; 338 :127379 - 32.
Bhowmik S, Panua R, Kumar Ghosh S, Debroy D, Paul A. A comparative study of artificial intelligence based models to predict performance and emission characteristics of a single cylinder diesel engine fueled with diesosenol. Journal of Thermal Science and Engineering Applications. 2018; 10 (4):041004 - 33.
Bhowmik S, Paul A, Panua R, Ghosh SK, Debroy D. Artificial intelligence based gene expression programming (GEP) model prediction of diesel engine performances and exhaust emissions under Diesosenol fuel strategies. Fuel. 2019; 235 :317-325 - 34.
Sharma P. Gene expression programming-based model prediction of performance and emission characteristics of a diesel engine fueled with linseed oil biodiesel/diesel blends: An artificial intelligence approach. Energy Sources, Part A: Recovery, Utilization, and Environmental Effects. 2020; 2020 :1-15 - 35.
Yıldırım S, Tosun E, Çalık A, Uluocak İ, Avşar E. Artificial intelligence techniques for the vibration, noise, and emission characteristics of a hydrogen-enriched diesel engine. Energy Sources, Part A: Recovery, Utilization, and Environmental Effects. 2019; 41 (18):2194-2206 - 36.
Viana DP, de Sá Só Martins DH, de Lima AA, Silva F, Pinto MF, Gutiérrez RH, et al. Diesel engine fault prediction using artificial intelligence regression methods. Machines. 2023; 11 (5):530 - 37.
Fernoaga V, Sandu V, Balan T. Artificial intelligence for the prediction of exhaust back pressure effect on the performance of diesel engines. Applied Sciences. 2020; 10 (20):7370 - 38.
Karimmaslak H, Najafi B, Band SS, Ardabili S, Haghighat-Shoar F, Mosavi A. Optimization of performance and emission of compression ignition engine fueled with propylene glycol and biodiesel–diesel blends using artificial intelligence method of ANN-GA-RSM. Engineering Applications of Computational Fluid Mechanics. 2021; 15 (1):413-425 - 39.
Ghaderi M, Javadikia H, Naderloo L, Mostafaei M, Rabbani H. Analysis of noise pollution emitted by stationary MF285 tractor using different mixtures of biodiesel, bioethanol, and diesel through artificial intelligence. Environmental Science and Pollution Research. 2019; 26 :21682-21692 - 40.
Khurana S, Saxena S, Jain S, Dixit A. Predictive modeling of engine emissions using machine learning: A review. Materials Today: Proceedings. 2021; 38 :280-284 - 41.
Azeez OS, Pradhan B, Shafri HZ, Shukla N, Lee CW, Rizeei HM. Modeling of CO emissions from traffic vehicles using artificial neural networks. Applied Sciences. 2019; 9 (2):313 - 42.
Maino C, Misul D, Di Mauro A, Spessa E. A deep neural network based model for the prediction of hybrid electric vehicles carbon dioxide emissions. Energy and AI. 2021; 5 :100073 - 43.
Pillai R, Triantopoulos V, Berahas AS, Brusstar M, Sun R, Nevius T, et al. Modeling and predicting heavy-duty vehicle engine-out and tailpipe nitrogen oxide (NOx) emissions using deep learning. Frontiers in Mechanical Engineering. 2022; 8 :11 - 44.
Wang X, Zhang P, Gao W, Li Y, Wang Y, Pang H. Misfire detection using crank speed and long short-term memory recurrent neural network. Energies. 2022; 15 (1):300 - 45.
Liu B, Zhao C, Zhang F, Cui T, Su J. Misfire detection of a turbocharged diesel engine by using artificial neural networks. Applied Thermal Engineering. 2013; 55 (1-2):26-32 - 46.
Ofner AB, Kefalas A, Posch S, Geiger BC. Knock detection in combustion engine time series using a theory-guided 1-D convolutional neural network approach. IEEE/ASME Transactions on Mechatronics. 2022; 27 (5):4101-4111 - 47.
Netzer C, Seidel L, Pasternak M, Klauer C, Perlman C, Ravet F, et al. Engine knock prediction and evaluation based on detonation theory using a quasi-dimensional stochastic reactor model (No. 2017-01-0538). In: SAE Technical Paper. USA: SAE; 2017 - 48.
Hoblos G, Benkaci M. A model-free diagnosis approach for intake leakage detection and characterization in diesel engines. Machines. 2015; 3 (3):157-172 - 49.
Mariani F, Grimaldi CN, Sgatti S, De Cesare M, Battistoni M. Artificial intelligence methodologies for oxygen virtual sensing at diesel engine intake (No. 2012-01-1153). In: SAE Technical Paper. USA: SAE; 2012 - 50.
Sujesh G, Ramesh S. Modeling and control of diesel engines: A systematic review. Alexandria Engineering Journal. 2018; 57 (4):4033-4048 - 51.
Naradasu KR, Jyothirmai S, Ramesh R. Towards artificial intelligence based diesel engine performance control under varying operating conditions using support vector regression. Thermal Science. 2013; 17 (1):167-178 - 52.
Malikopoulos AA, Assanis DN, Papalambros PY. Real-time self-learning optimization of diesel engine calibration. Journal of Engineering for Gas Turbines and Power. 2009; 131 (2):022803-022810