Parameters and definition related to energy fluxes obtained from OJIP-test.
Abstract
Plant diseases are a major threat to food security, causing drastic alterations in plant metabolism upon infection by pathogens. This often results in decreased biomass accumulation, slowed growth rates, and diminished yield components. Pathogens, through various lifestyles such as biotrophic, necrotrophic, and hemibiotrophic, disrupt photosynthesis, the primary metabolic process, via functional and structural damages. Furthermore, the CO2 assimilation in plants is severely altered by pathogens regardless of their lifestyles. Photosynthetic determinations allow us to establish a perspective about the physiological impairment caused by pathogens related to alterations in the CO2 flow from the atmosphere to carboxylation sites, stomatal limitations, and photosynthetic performance of photosystem II (PSII). From the changes in the energy, dissipation is possible to establish the functional status of the photochemistry machinery under stress conditions. For the above, chlorophyll a fluorescence (CF) and CF imaging (CFI) arose as a method highly sensible to determine the damage caused by pathogens in plants. This review shows a practical perspective on CF tools using visual method and rapid fluorescence induction kinetics (OJIP-test), for disease detection associated with plant-pathogen interaction studies from the physiological viewpoint, their implications for plant pathology research, applications for the plant phenotyping field, and biotic stress detection.
Keywords
- photosynthesis
- pathogen
- plant-pathogen interaction
- primary metabolism
- plant phenotyping
1. Introduction
The photosynthetic process is one of the most important metabolic pathways through which plants transform the sunlight into chemical energy obtaining carbohydrates to be used in other metabolic events [1]. In plants, the sunlight energy may be dissipated by photochemistry (photosynthesis light reactions), chlorophyll fluorescence (photon re-emission), and heat (Figure 1) [2]. Metabolic process of photosynthesis has been studied in two stages: (i) light reactions: these processes allow the solar energy contained in photons of sunlight to be processed in the membranes of chloroplasts to produce adenosine triphosphate (ATP) and nicotinamide adenine dinucleotide phosphate (NADPH) and (ii) the Calvin-Benson cycle, which produces triose-P and, as a result, the recovery of ribulose 1,5-bisphosphate (RuBP), in which how CO2 is reduced [3, 4]. Chlorophyll fluorescence occurs when the electron transfer from a chlorophyll molecule at the reaction center of the photosystem II (PSII) to a plastoquinone molecule within the plastoquinone transporter, results in fluorescence emission [2]. Finally, the energy cannot be dissipated by photochemistry or fluorescence, and it is dissipated by heat emission [5, 6]. Under this perspective, plants utilize the sun’s energy mainly to supply the demands of the primary metabolism; however, external (stresses) and intrinsic (diffusional and biochemical limitations) factors related to the photosynthetic process induce alternative pathways such as fluorescence and heat emission to help in the energy dissipation process to reduce functional and structural damages in the plant cell derivatives of energy accumulation [2].
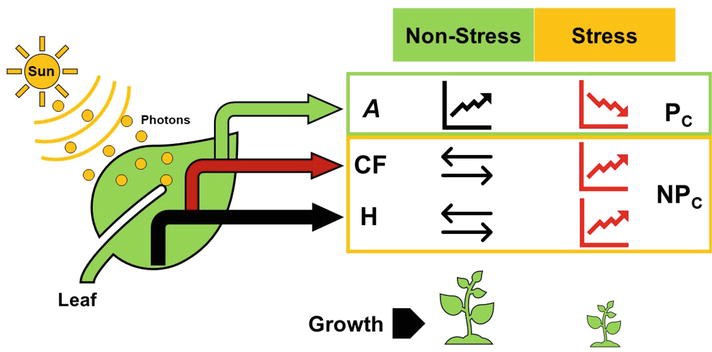
Figure 1.
Energy dissipation pathways (photochemistry (
To study the photosynthesis, several methodologies have been developed involving determinations based on changes in CO2 and water vapor differential concentrations using infrared sensors (infrared gas analyzer (IRGA)) through which it is possible calculate the leaf gas exchange parameters such as net CO2 assimilation rate (
On the other hand, the chlorophyll a fluorescence (CF) has been a non-invasive and non-destructive method successful to study photosynthetic responses from plants under different conditions [11]. Currently, the CF method is applied in diverse fields, especially for rapid diagnosis of abiotic or stress biotic [12, 13] based on changes in the energy dissipation magnitude in the photochemical reactions [10]. Basically, the CF method has been developed from “Kautsky’s effect,” which was studied when the photosynthetic sample was transferred from the dark to the light, the CF yield increased over a time span of about 1 second. This effect led to increases in the CF yield with a consequent reduction of electron acceptors in the photosynthetic pathway inducing quinone A (QA) reduction due to one electron reception which must be transferred to quinone B (QB), ensuring the linear electron flow [5]. Therefore, variations in CF characteristics can be indicative of various stresses, including nutrient deficiencies, water deficit, pathogen infections, and high light intensity [2]. By monitoring the CF, scientists, agronomists, and other professionals can gain insights into plant performance and make informed decisions regarding crop management and stress mitigation strategies. In this sense, the CF can be used as a diagnostic tool for assessing plant health and stress responses.
For the plant pathology field, the CF tool has been used for the detection of changes in the energy dissipation pattern in several pathosystems related to fungi, viruses, bacteria, and oomycetes, for example,
The use of these devices has allowed crop monitoring on the field and under controlled conditions, plant health management, and ecosystem dynamics [23]. Additionally, the CF imaging technique enable the spatial and temporal visualization of photosynthetic activity, allowing for the assessment of plant performance and the detection of heterogeneity in responses [6]. The integration of the CF with other “omics” technologies further enhances our understanding of photosynthetic regulation and stress responses at the molecular, metabolic, genomic, and phenomic level [24]. Overall, the CF technique plays a crucial role in advancing our knowledge of plant function and resilience. In this sense, the aim of the review was describing the use of the CF as a technique of determination of biotic stress in the plant pathology field.
2. Chlorophyll a fluorescence: method and application
The CF has been used as a technique in photosynthesis research, providing valuable information on the structure and function of the photosynthetic apparatus [10, 12]. In this sense, the photosynthetic response can be estimated by fluorescence changes which study through two types of CF measurement such as continuous excitation and pulsed excitation [5].
Firstly, a method uses pulse amplitude modulation (PAM). The PAM method measures CF induced by high-frequency, modulation pulses (MP) at different stages of the system dynamics driven by saturation pulses (SP) or actinic light (AL) [25, 26]. Thus, the relative yield of fluorescence can be measured in the presence of illumination or can be conducted at field condition [5]. Fundamentally, the CF method determines values associated with basal (F0 and F0′) and maximal (Fm and Fm′) fluorescence on dark and light-adapted leaves from which it has been possible to derive parameters to estimate specific responses of the energy dissipation through the photochemical pathway [5]. Experimentally, F0, F0′, Fm, and Fm′ are obtained from a sequence of light supplying to different intensities which simulated the change from darkness to light adaptation through photosynthetic excitation (Figure 2). For obtaining F0, the dark-adapted leaf is illuminated with weak, modulated measuring pulse (L1 = 0.03 μmol m−2 s−1). Immediately, a light saturate pulse (SP = 8000 μmol m−2 s−1) is applied for 0.8 s to ensure maximal fluorescence emissions (Fm). Subsequently, on light-adapted leaves, the steady-state fluorescence yield (Ft) is measured following a saturating light pulse (SP = 8000 μmol m−2 s−1, 0.8 s) that was applied to achieve the light-adapted maximal fluorescence (Fm′). The actinic light (AL) is then turned off and far-red illumination was applied (2 μmol m−2 s−1) to measure the light-adapted basal fluorescence (F0′) [7].
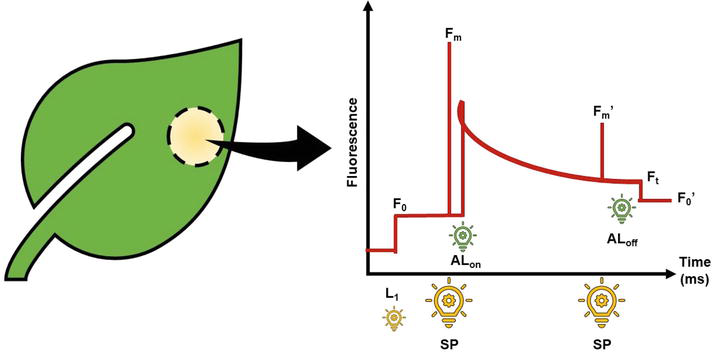
Figure 2.
Fluorescence determination routine. Lowing intensity light (L1); light saturated pulse (SP); actinic light on (ALon) and off (ALoff); basal (F0) and maximal (Fm) fluorescence in dark-adapted leaf; basal (F0′) and maximal (Fm′) fluorescence in light-adapted leaf; fluorescence level immediately before the SP (Ft). Figure adapted from Baker [
The second method is based on the increase in the CF yield observed when leaves pass through dark to light in a short time period. This induction and fast dynamic changes of CF occur when dark-adapted samples are exposed to light known as Kautsky’s effect, as explained above [27], and it is observed as a polyphasic curve, giving information about the efficiency of electron transport through PSII [28, 29]. The CF variations on the OJIP induction curve are also well-known as the OJIP-test. From the O-step to the J-step, it reflects high-order nonlinear dynamics of PSII resulting from light excitation, energy transfer, energy dissipation, and photochemical reactions [25], see Table 1 for more details. The technique is based on the theory that energy flux through PSII reaction centers can be quantified to provide information about structure and function of the photosynthetic apparatus [33].
Technical fluorescence parameters | |
---|---|
Area | Area between fluorescence curve and FM |
M0 | Slope of the curve at the origin of the fluorescence rise. It is a measure of the rate of the primary photochemistry. |
Vj | Relative variable fluorescence at 2 ms |
Vi | Relative variable fluorescence at 30 ms |
SM = Area/FV | Normalized total complementary area above the OJIP transient (reflecting multiple-turnover |
N | The number of rotations: number of |
ABS | The photon flux absorbed by the antenna of PSII units |
TR | The portion of ABS trapped by the active PSII units that leads to QA reduction |
DI | The portion of ABS dissipated in PSII antenna in process other than trapping |
ET | The energy flux associated with the electron transport from QA− to the intersystem electron acceptors |
RE | The energy flux associated with the electron transport from QA− to the final electron acceptors of PSI |
ϕPo = TR0/ABS | Maximum quantum yield of primary photochemistry (at t = 0) ϕPo = (FM – F0)/FM |
ψ | Probability (at t = 0) that a photon trapped by the PSII reaction center enters the electron transport chain ψ |
ϕ | Quantum yield of electron transport (at t = 0) ϕ |
ϕ | Quantum yield (at t = 0) of energy dissipation. ϕ |
ϕ | Quantum yield of an electron transport from QA− to the final electron acceptors of PSI. ϕRo = [(1 – F0/FM) × (1 – Vi)] |
δ | Efficiency with which an electron from PQH2 is transferred to final PSI acceptors δ |
ABS/RC | Effective antenna size of an active reaction center (RC). Expresses the total number of photons absorbed by chlorophyll molecules of all RCs divided by the total number of active RCs ABS/RC = (M0/Vj)/ϕPo |
TR0/RC | Maximal trapping rate of PSII. Describes the maximal rate by which an excitation is trapped by the RC TRo/RC = M0/Vj |
ET0/RC | Electron transport in an active RC ETo/RC = (M0/Vj)/ψ |
DI0/RC | Dissipated energy flux per RC DI0/RC = ABS/RC – TR0/RC |
ABS/CS0 | Absorption per cross-section (CS) ABS/CS0 ≈ F0 and ABS/CSM ≈ FM |
TR0/CS | Trapping per cross-section TR0/CS = (TR0/ABS) × (ABS/CS) |
ET0/CS | Electron transport per cross-section ET0/CS = (ET0/ABS) × (ABS/CS) |
RE0/CS | Electron flux from QA− to final PSI acceptors per cross section of PSI RE0/CS = (RE0/ABS) × (ABS/CS) |
PIABS | Performance index on adsorption basis PIABS = (RC/ABS) × [ϕPo/(1 – ϕPo)] × [ψ |
PIABS, total | Total performance index on absorption basis PIABS, total = PIABS × [δR0/(1 – δR0)] |
PICS | Performance index on cross section basis PICS = PIABS × (ABS/CS) |
PICS, total | Total performance index on cross section basis PICS, total = PIABS, total × (ABS/CS) |
Several CF parameters are commonly used to evaluate the performance of the photosynthetic apparatus, such as the maximum quantum yield of photosystem II (PSII), often represented as the Fv/Fm, provides information about the maximum efficiency of PSII photochemistry [5]. The effective quantum yield of PSII (ΦPSII) reflects the actual efficiency of light energy conversion in PSII [2] and non-photochemical quenching (NPQ) that represents the dissipation of excess light energy as heat, thereby protecting the photosynthetic machinery from photodamage [34]. The increases in non-photochemical quenching (measured as NPQ and/or
Parameter | Description | Formula/interpretation |
---|---|---|
F0; F0′ | Basal or initial fluorescence from dark and light-adapted leaves, respectively | Level of florescence when all PSII center open (oxidated status) |
Fm; Fm′ | Maximal fluorescence from dark and light-adapted leaves, respectively | Level of florescence when all PSII center closed (reduced status) |
Fv; Fv′ | Variable fluorescence from dark and light-adapted leaves, respectively | Fm – F0 It is value associated with the PSII capacity to perform photochemistry |
Fv/Fm | Maximum quantum yield of PSII | (Fm – F0)/Fm Maximum efficiency at which light absorbed by PSII is utilized to reduce QA |
Fv′/Fm′ | Capturing efficiency of the excitation energy by the open PSII reaction centers | (Fm′ – F0′)/Fm′ |
Coefficient for photochemical quenching | (Fm′– Ft)/(Fm′ – F0′) Actual photochemical capacity | |
Coefficient for photochemical quenching | 1 – [(Fm′ – F0′)/Fm – F0] Non-photochemical process activation during the light period | |
ΦPSII | Actual quantum yield of PSII electron transport | (Fv′/Ft)/Fm′ |
NPQ | Non-photochemical quenching | (Fm – Fm′) – 1 Apparent heat loss rate from PSII |
ETR = J | Rate of electron transport | PPFD × ФPSII × ƒ × α |
Table 2.
Chlorophyll fluorescence parameters used for studies of photosystem II (PSII) photochemistry.
PPFD is the photosynthetic photon flux density. ƒ is a factor that accounts for the partitioning of energy between PSII and PSI and is assumed to be 0.5, which indicates that the excitation energy is distributed equally between the two photosystems; and α is the leaf absorbance by the photosynthetic tissues and is assumed to be 0.84.
The CF parameters obtained from measurements can provide valuable information about the plant’s photosynthetic efficiency, tolerance to stress (abiotic and biotic), or normal physiological condition [6, 35]. Therefore, measuring changes in fluorescence parameters such as Fv/Fm, ΦPSII, and NPQ provides insights into the functionality and regulation of PSII and can indicate early stress detection [34]. In this context, the NPQ represents the protective mechanism employed by plants to dissipate excess absorbed light energy as heat, reducing the potential for photodamage [22]. In addition, the NPQ is influenced by factors such as light intensity, temperature, and the availability of energy sinks within the plant [36]. In practice, the NPQ determination requires leaves’ full adaptation to darkness in such a way it reflects the energy dissipation capacity in excess, especially under stress conditions [6]. Recently Tietz et al. [37] proposed the characterization a new set of parameters, NPQ (T), that can be used without dark adaptation and can be rapidly applied during steady-state illumination, potentially even under field conditions.
On the other hand, in the last years, with the developments in optical sensors, the CF imaging (CFI) technique emerged as a powerful tool to study plants under different growth conditions, especially stress factors. In this case, the fluorescence spectral considers the wavelength from UV to infrared, allowing the examination of the spectral region’s keys for the plant’s physiology responses. In addition, the physical characteristic is related to the emission of fluorescence when the energy is absorbed in the UV region, invisible to the human eye, and posterity is emitted in the visible region and can facilitate obtaining images [38]. Basically, a CFI system utilizes a camera with a long-pass filter, while hindering shorter wavelengths. From the images captured, they are segmented and CF parameters are calculated for each pixel, finishing with the visualization of CF images [39]. With this technique, the obtaining of images from each parameter associated with the CF such as Fv/Fm, ΦPSII, NPQ , and ETR allows the visual exploration of changes in these parameters per area unit [40] and spatial heterogeneity distribution of CF [41]. It has been such a sensibility in this technique that the CFI sensors have been coupled to plant phenotyping systems, obtaining interesting results on indoor and outdoor platforms [10].
3. Photosynthetic alterations by pathogen infection
Under biotic stress, the CO2 assimilation in plants is severely altered by pathogens infection regardless of their lifestyles (e.g., biotrophic, necrotrophic, and hemibiotrophic) [1, 42]. The biotrophic pathogens get to grow and feed on living plant tissue colonizing plant cells undetected through mechanisms that suppress the plant responses to infection [43]. The biotrophic strategy is considered obligatory parasitism, where the pathogen establishes a molecular and physiological manipulation of the plant cell, and this way it obtains the energy for the growth, such as the causal pathogens associated with rust (e.g.,

Figure 3.
Leaf symptoms caused by biotrophic (A;
During the plant-pathogen interactions, plants infected by pathogens have reported reductions in CO2 assimilation, stomatal dynamic alterations (stomatal closure), chronic inhibition of the photosystems, higher dissipation of energy by chlorophyll a fluorescence (non-photochemical mechanisms), alterations in the concentration of photosynthetic pigments, chloroplasts structural damage, losses in leaf area index, changes in the source-sink ratio, and changes in the profiles of genes, proteins, and metabolic related to photosynthesis [1, 8, 9, 52]. The pathogen infection in the foliar tissue causes loss in the sunlight conduction capacity due to the cellular damage affecting the photosynthetic activity and consequent development of chlorotic and necrotic zones product of the action of hydrolytic enzymes, non-host selective toxins, and reactive oxygen species (ROS) [1, 53]. While, the plant cell after detection of the pathogen, genes related to photosynthesis are transcript down-regulated to protect the photosynthetic apparatus against the oxidative damage [1, 42].
CO2 assimilation by the photosynthetic process is a permanent source of primary metabolism, and their products are the main energetic nutrient for pathogens colonizing plant tissues, as well as molecules related to metabolic pathways from secondary metabolism [1, 54]. Studies performed in the last 20 years have allowed understanding how pathogens reprogram the metabolic flux of the carbohydrate’s metabolism in detriment of the plant growth and yield demands [1, 8, 42, 54]. From the metabolic perspective, several pathogens rapidly metabolize sucrose through invertase enzymes which hydrolyze sucrose to fructose and glucose increasing the hexoses pool [8, 14, 55]. Metabolites such as fructose, glucose, and hexoses are energetic substrates for other metabolic pathways more complex that under biotic stress those molecules become a nutrient source for pathogens; however, alterations in the sugar concentrations induce the expression of defense genes and cell death considering that sugars act as signaling molecules to regulate the expression of some genes [56]. In the case of foliar pathogens, increases on the invertases activities cause changes in the source-sink relationship dynamic [14, 23, 57].
On the other hand, studies have reported that the damage caused by pathogens on leaves led to significant reductions in the net CO2 assimilation rate (
Studies focused on energy dissipation through CF have allowed an understanding of the physiological events that happened during the asymptomatic and symptomatic disease phases. It is well-known that the Fv/Fm index is the most extensively utilized chlorophyll a fluorescence parameter stress level determination of abiotic and biotic factors [2]. Under non-stressed conditions, healthy leaves show Fv/Fm values around 0.80–0.83, while inferior Fv/Fm values indicate that a proportion of photosystem II (PSII) reaction centers have been harmed, which induces the photo-inhibitory effect on the photosynthetic machinery and is often led to oxidative stress on plants [63, 64]. In practice, the Fv/Fm parameter is a powerful tool for judging plant photochemical capacity because it acts as a stress indicator for plants; in other words, Fv/Fm measures the operational capacity of the leaf to perform photosynthesis [2, 5, 63, 64].
In the plant pathology field, the use of Fv/Fm has been efficient to measure the pathogen infection damage on the photosynthetic response depending on pathogen lifestyles [10]. However, CF parameters related to photochemical and non-photochemical energy dissipation also have showed interesting findings in plants infected. For instance, in coffee plants with rust symptoms, the values of the yield for dissipation by down-regulation [Y(NPQ) = (Fs/Fm′) – (Fs/Fm)] and the yield for other non-photochemical (non-regulated) losses [Y(NO) = Fs/Fm] were lower and higher, respectively, for the inoculated plants compared to non-inoculated plants, suggesting that
In the plant resistance field, the photosynthetic damage leads to intrinsic reductions in the defense capacity against the pathogen, and it can reach a higher intensification when abiotic stress factors such as drought, high temperature, or nutritional deficiency/excess participate along the biotic interaction [8, 67, 68]. As known, studies demonstrated that pathogens secret their effectors for linking to receptor specifics in chloroplasts looking for a reduction drastic in the ROS controlling the plant defense mechanisms, suggesting the crucial role of the photosynthetic machinery in the basal plant resistance against pathogen infection [69]. In this sense, the manipulation of photosynthetic activity using photosynthesis inhibitor-specific (DCMU) in wheat cultivars with differential basal resistance to
In short, photosynthesis is affected by pathogen infections, and their effects led to reductions in CO2 assimilation, losses in growth, and crop yield, suggesting that just like abiotic stress, biotic factors threaten food production, as has been reported from the famine in Ireland at XVIII caused by the potato blight epidemic. For the above reasons, deep knowledge about the pathogen strategies to cause disease in plants and how they affect plant metabolism is crucial to designing management alternatives in all fields of agronomy.
4. CF tool to study the plant-pathogen interaction
The use of CF tools in recent years has revealed the utility of this tool from a plant pathology perspective, and CF imaging (CFI) techniques have been used in several plant pathology interactions. However, the equipment for CFI can be expensive, and it is necessary to handle due to the dark adaptation, increasing the time required with a large numbers of plants to test [6]. Today, portable instruments are commercially available being used at field conditions. For instance, CF using the OJIP-test, that is, an induction curve from the O-step to the J-step reflects high-order nonlinear dynamics of PSII resulting from light excitation, energy transfer, energy dissipation, and photochemical reactions (Table 1) [33]. As a result, OJIP-test is fast, non-invasive, and sensitive technic [6, 11], becoming an alternative for physiological and plant pathogen studies. Also, this technique allows screening and phenotyping large number of plants (e.g., approximately 500 plants per day) under field conditions, as was reported by Ajigboye et al. [71].
Both techniques offer important information on the PSII damage caused by the infection of pathogens in plants; however, CFI has been more frequently used to detect CF changes in response to foliar diseases in different genotypes due to their visual advantages [8, 40, 72]. In this context, CFI has been used to screen resistant wheat genotypes as well as the early detection of leaf rust caused by
On the other hand, Fv/Fm and Y(II) parameters were sensible on coffee leaves inoculated with
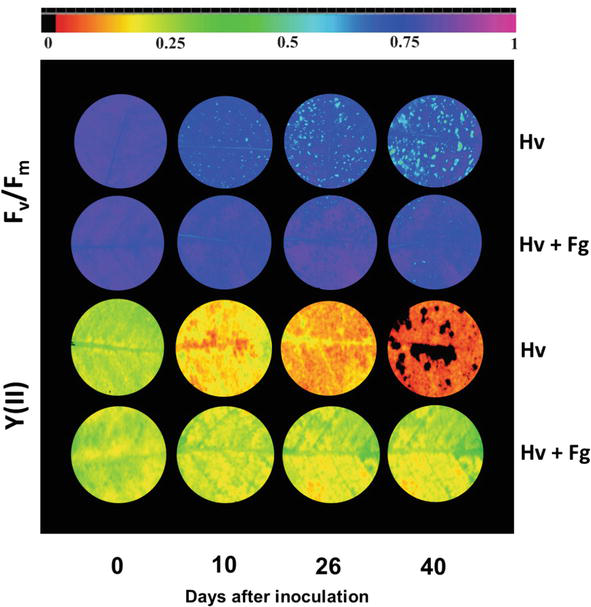
Figure 4.
The maximum quantum yield of PSII (Fv/Fm) and the effective PSII quantum yield [Y(II)] images in coffee plants infected with
The combination of the CFI with other techniques allows an important way to study plant-pathogen interaction. In a study, combining CFI and leaf thermography from thermal infrared (TIR) imaging, among sweet potatoes infected by Sweet potato feathery mottle virus (SPFMV, genus Potyvirus) and Sweet potato chlorotic stunt virus (SPCSV, genus Crinivirus), demonstrated that the Fv/Fm and
The detection of some diseases in the most cases results in a difficult task due to the complexity between symptomatology observed. In this respect, the combination of CFI and ROS activities detects brown spot and anthracnose in cucumber plants. The difference of the activity levels of the ROS-scavenging enzymes and CFI parameters discriminated cucumber diseases [81]. Likewise, an important disease in citrus such as Citrus Huanglongbing (HLB) had been rapidly detected with high accuracy with the combination of reflectance images and multicolor fluorescence images, which relate to photosynthesis and secondary metabolites [82]. The study demonstrated that the combination of the techniques can effectively detect HLB disease in different infected statuses and cultivars, making it a promising tool for high-throughput HLB disease detection in citrus orchards. Currently, the increases in the interest on artificial intelligent (IA) in the recent years demonstrated the versatility of the CFI for the development of possible tools that discriminate biotic stress. Recently, Sapoukhina et al. [83] proposed a new methodology to develop a data set to generate images from CFI of plants infected by
On the other hand, the CF by OJIP-test has been used in several studies to determinate the effects over PSII performance. In this case, Ajigboye et al. [11] using CF determines that existing changes related to the maximum efficiency of PSII photochemistry (Fv′/Fm′), as well as flux of dissipated (DIo/RC), trapped (TRo/RC), or absorbed (ABS/RC) energy per active reaction centers during the attack of necrotrophic pathogens such as
In another study, the use of OJIP-test showed that DIo/RC parameter is the best indicator for the diagnosis of
The CF methodology has been successful in several studies to determine the effect of fungicides on PSII activity during disease control, as mentioned above (Figure 4). For instance, the use a fungicide such as isopyrazam and epoxiconazole increased PSII efficiency, ameliorate biomass and grain yield, and reduce disease pressure. Also, the two fungicides increased the efficiency of PSII photochemistry (Fv’/Fm′), as well as improved photosynthetic gas exchange and increased rates of electron transport [71]. In another study, the application of dimethyl sulfoxide (DMSO) in
Additionally, this tool can be used for phenotyping in breeding programs. In this context, a study conducted by Suarez et al. [89] using CF determination showed that common bean lines induced by biotic stress can be classified as resistant or susceptible phenotypes according to the changes in F0, Fm, and consequent alteration in Fv/Fm. In barley genotypes infected with
5. Conclusions
The CF is a versatile technique that offers valuable insights into the efficiency and performance of the photosynthetic apparatus. With advancements in methodology and instrumentation, the CF has become an indispensable tool in plant science research, specifically in the plant pathology field.
The measurement setup, parameters obtained, and data interpretation are crucial aspects of the Chl a fluorescence methodology. Researchers employ specialized fluorometers, measure fluorescence parameters such as Fv/Fm, ΦPSII, and NPQ , and interpret the obtained data in conjunction with other physiological measurements for biotic stress detection. However, other parameters have shown high sensitivity depending on the pathosystem, so more studies are necessary for confirming responses and developing new research approaches to improve our knowledge about pathogen infection strategies. Plant physiologists and plant pathologists, as well as plant breeders, hope that, in the next few years, new advances in CF technology will allow a higher understanding of plant responses under pathogen infection, as well as become a powerful tool to support the disease management in issues related to fast detection, evaluation of efficiency in control strategies, and also improving the selection criteria for resistant phenotypes to disease within plant breeding programs.
Acknowledgments
The authors are thankful to Fondo de Investigación para la Agrobiodiversidad, Semillas y Agricultura sustentable (FIASA), grant number FIASA-CA-2023-010.
References
- 1.
Bolton MD. Primary metabolism and plant defense—fuel for the fire. Molecular Plant-Microbe Interactions. 2009; 22 (5):487-497. DOI: 10.1094/MPMI-22-5-0487 - 2.
Baker NR. Chlorophyll fluorescence: A probe of photosynthesis in vivo. Annual Review of Plant Biology. 2008; 59 :89-113. DOI: 10.1146/annurev.arplant.59.032607.092759 - 3.
Spreitzer RJ, Salvucci ME. Rubisco: Structure, regulatory interactions, and possibilities for a better enzyme. Annual Review of Plant Biology. 2002; 53 (1):449-475. DOI: 10.1146/annurev.arplant.53.100301.135233 - 4.
Leister D. Enhancing the light reactions of photosynthesis: Strategies, controversies and perspectives. Molecular Plant. 2023; 14 :4-22. DOI: 10.1016/j.molp.2022.08.005 - 5.
Maxwell K, Johnson GN. Chlorophyll fluorescence-a practical guide. Journal of Experimental Botany. 2000; 51 (345):659-668. DOI: 10.1093/jexbot/51.345.659 - 6.
Murchie EH, Lawson T. Chlorophyll fluorescence analysis: A guide to good practice and understanding some new applications. Journal of Experimental Botany. 2013; 64 (13):3983-3998. DOI: 10.1093/jxb/ert208 - 7.
Aucique-Perez CE, Rodrigues FÁ, Moreira WR, DaMatta FM. Leaf gas exchange and chlorophyll a fluorescence in wheat plants supplied with silicon and infected with Pyricularia oryzae . Phytopathology. 2014;104 (2):143-149. DOI: 10.1094/PHYTO-06-13-0163-R - 8.
Aucique-Pérez CE, Rios VS, Neto LBC, Rios JA, Martins SCV, Rodrigues FÁ. Photosynthetic changes in wheat cultivars with contrasting levels of resistance to blast. Journal of Phytopathology. 2020; 168 (11-12):721-729. DOI: 10.1111/jph.12952 - 9.
Barón M, Flexas J, Delucia EH. Photosynthetic responses to biotic stress. In: Flexas J, Loreto F, Medrano H, editors. Terrestrial Photosynthesis in a Changing Environment. A Molecular, Physiological and Ecological Approach. Cambridge, UK: Cambridge University Press; 2012. pp. 331-350 - 10.
Pérez-Bueno ML, Pineda M, Barón M. Phenotyping plant responses to biotic stress by chlorophyll fluorescence imaging. Frontiers in Plant Science. 2019; 10 :1135. DOI: 10.3389/fpls.2019.01135 - 11.
Ajigboye OO, Bousquet L, Murchie EH, Ray RV. Chlorophyll fluorescence parameters allow the rapid detection and differentiation of plant responses in three different wheat pathosystems. Functional Plant Biology. 2016; 43 (4):356-369. DOI: 10.1071/FP15280 - 12.
Govindjee. Chlorophyll a fluorescence: A bit of basics and history. In: Chlorophyll a fluorescence: A signature of photosynthesis. Dordrecht: Springer; 2004. pp. 1-41. DOI: 10.1007/z978-1-4020-3218-9_1 - 13.
Schreiber U. Pulse-amplitude-modulation (PAM) fluorometry and saturation pulse method: An overview. Chlorophyll a Fluorescence. 2004; 19 :279-319. DOI: 10.1007/978-1-4020-3218-9_11 - 14.
Rios JA, Aucique-Pérez CE, Debona D, Cruz Neto LB, Rios VS, Rodrigues FA. Changes in leaf gas exchange, chlorophyll a fluorescence and antioxidant metabolism within wheat leaves infected by Bipolaris Sorokiniana . Annals Applied Biology. 2017;170 (2):189-203. DOI: 10.1111/aab.12328 - 15.
Bauriegel E, Herppich WB. Hyperspectral and chlorophyll fluorescence imaging for early detection of plant diseases, with special reference to fusarium spec. infections on wheat. Agriculture. 2014; 4 (1):32-57. DOI: 10.3390/agriculture4010032 - 16.
Bürling K, Hunsche M, Noga G. Use of blue–green and chlorophyll fluorescence measurements for differentiation between nitrogen deficiency and pathogen infection in winter wheat. Journal of Plant Physiology. 2011; 168 (14):1641-1648. DOI: 10.1016/j.jplph.2011.03.016 - 17.
Scholes JJD, Rolfe SA. Photosynthesis in localised regions of oat leaves infected with crown rust ( Puccinia Coronata ): Quantitative imaging of chlorophyll fluorescence. Planta. 1996;199 (4):573-582. DOI: 10.1007/BF00195189 - 18.
Brabandt H, Bauriegel E, Gärber U, Herppich WB. ФPSII and NPQ to evaluate Bremia lactucae -infection in susceptible and resistant lettuce cultivars. Scientia Horticulturae. 2014;180 :123-129. DOI: 10.1016/j.scienta.2014.10.012 - 19.
Rodríguez-Moreno L, Pineda M, Soukupová J, Macho AP, Beuzón CR, Barón M, et al. Early detection of bean infection by Pseudomonas syringae in asymptomatic leaf areas using chlorophyll fluorescence imaging. Photosynthesis Research. 2008;96 (1):27-35. DOI: 10.1007/s11120-007-9278-6 - 20.
Christov I, Stefanov D, Velinov T, Goltsev V, Georgieva K, Abracheva P, et al. The symptomless leaf infection with grapevine leafroll associated virus 3 in grown in vitro plants as a simple model system for investigation of viral effects on photosynthesis. Journal of Plant Physiology. 2007; 164 (9):1124-1133. DOI: 10.1016/j.jplph.2005.11.016 - 21.
Montero R, Pérez-Bueno ML, Barón M, Florez-Sarasa I, Tohge T, Fernie AR, et al. Alterations in primary and secondary metabolism in Vitis vinifera ‘malvasía de Banyalbufar’upon infection with grapevine leafroll-associated virus 3. Physiology Plant. 2016;157 (4):442-452. DOI: 10.1111/ppl.12440 - 22.
Guidi L, Lo Piccolo E, Landi M. Chlorophyll fluorescence, photoinhibition and abiotic stress: Does it make any difference the fact to be a C3 or C4 species? Frontiers in Plant Science. 2019; 10 :174. DOI: 10.3389/fpls.2019.00174 - 23.
Roháček K, Soukupová J, Barták M. Chlorophyll fluorescence: A wonderful tool to study plant physiology and plant stress. In: Schoefs B, editor. Plant cell Compartments- Selected Topics. Kerala India: Research Signpost; 2008. pp. 41-104 - 24.
Großkinsky DK, Syaifullah SJ, Roitsch T. Integration of multi-omics techniques and physiological phenotyping within a holistic phenomics approach to study senescence in model and crop plants. Journal of Experimental Botany. 2018; 69 (4):825-844. DOI: 10.1093/jxb/erx333 - 25.
Guo Y, Tan J. Recent advances in the application of chlorophyll a fluorescence from photosystem II. Photochemestry Photobiology. 2015; 91 (1):1-14. DOI: 10.1111/php.12362 - 26.
Schreiber U. Chlorophyll fluorescence: New instruments for special applications. In: Garab G, editor. Photosynthesis: Mechanisms and Effects, Proceedings of the XIth International Congress on Photosynthesis; August 17-22; Budapest, Hungary. Vol. I–V. Dordrecht: Springer; 1998. pp. 4253-4258. DOI: 10.1007/978-94-011-3953-3_984 - 27.
Stirbet A. On the relation between the Kautsky effect (chlorophyll a fluorescence induction) and photosystem II: Basics and applications of the OJIP fluorescence transient. Journal of Photochemistry and Photobiology B: Biology. 2011; 104 (1-2):236-257. DOI: 10.1016/j.jphotobiol.2010.12.010 - 28.
Strasser RJ, Tsimilli-Michael M, Srivastava A. Analysis of the chlorophyll a fluorescence transient. In: Chlorophyll a Fluorescence. Dordrecht: Springer; 2004. pp. 321-362. DOI: 10.1007/978-1-4020-3218-9_12 - 29.
Stirbet A, Govindjee. Chlorophyll a fluorescence induction: A personal perspective of the thermal phase, the J–I–P rise. Photosynthesis Research. 2012; 113 :15-61. DOI: 10.1007/s11120-012-9754-5. DOI: 10.1007/s11120-012-9754-5 - 30.
Strasser RJ, Tsimilli-Michael M, Qiang S, Goltsev V. Simultaneous in vivo recording of prompt and delayed fluorescence and 820-nm reflection changes during drying and after rehydration of the resurrection plant Haberlea rhodopensis. Biochimica Biophysica Acta (BBA)-Bioenergetics. 2010; 1797 :1313-1326. DOI: 10.1016/j.bbabio.2010.03.008 - 31.
Stirbet A, Lazár D, Kromdijk J, Govindjee. Chlorophyll a fluorescence induction: Can just a one-second measurement be used to quantify abiotic stress responses? Photosynthetica. 2018; 56 :86-104. DOI: 10.1007/s11099-018-0770-3 - 32.
Bussotti F, Desotgiu R, Pollastrini M, Cascio C. The JIP test: A tool to screen the capacity of plant adaptation to climate change. Scandinavian Journal of Forest Research. 2010; 25 :43-50. DOI: 10.1080/02827581.2010.485777 - 33.
Strasser RJ, Srivastava A, Tsimilli-Michael M. The fluorescence transient as a tool to characterize and screen photosynthetic samples. Probing Photosynthesis: Mechanism, Regulation & Adaptation. 2000; 25 :445-483 - 34.
Kalaji HM, Schansker G, Brestic M, Bussotti F, Calatayud A, Ferroni L, et al. Frequently asked questions about chlorophyll fluorescence, the sequel. Photosynthesis Research. 2017; 132 :13-66. DOI: 10.1007/s11120-016-0318-y - 35.
Krause GH, Weis E. Chlorophyll fluorescence and photosynthesis: The basics. Annual Review of Plant Physiology and Plant Molecular Biology. 1991; 42 (1):313-349. DOI: 10.1146/annurev.pp.42060191.001525 - 36.
Nishiyama Y, Murata N. Revised scheme for the mechanism of photoinhibition and its application to enhance the abiotic stress tolerance of the photosynthetic machinery. Applied Microbiology and Biotechnology. 2014; 98 :8777-8796. DOI: 10.1007/s00253-014-6020-0 - 37.
Tietz S, Hall CC, Cruz JA, Kramer DM. NPQ (T): A chlorophyll fluorescence parameter for rapid estimation and imaging of non-photochemical quenching of excitons in photosystem-II-associated antenna complexes. Plant Cell & Environment. 2017; 40 (8):1243-1255. DOI: 10.1111/pce.12924 - 38.
Goodwin RH. Fluorescent substances in plants. Annual Review of Plant Physiology. 1953; 4 (1):283-304. DOI: 110.1146/annurev.pp.04.060153.001435 - 39.
Nedbal L, Whitmarsh J. Chlorophyll fluorescence imaging of leaves and fruits. In: Chlorophyll a Fluorescence: A Signature of Photosynthesis. Dordrecht: Springer; 2004. pp. 389-407. DOI: 10.1007/978-1-4020-3218-9_14 - 40.
Rolfe SA, Scholes JD. Chlorophyll fluorescence imaging of plant–pathogen interactions. Protoplasma. 2010; 247 (3-4):163-175. DOI: 10.1007/s00709-010-0203-z - 41.
Gorbe E, Calatayud A. Applications of chlorophyll fluorescence imaging technique in horticultural research: A review. Scientia Horticulturae (Amsterdam). 2012; 138 :24-35. DOI: 10.1016/j.scienta.2012.02.002 - 42.
Berger S, Sinha AK, Roitsch T. Plant physiology meets phytopathology: Plant primary metabolism and plant–pathogen interactions. Journal of Experimental Botany. 2007; 58 (15-16):4019-4026. DOI: 10.1093/jxb/erm298 - 43.
Vleeshouwers VGAA, Oliver RP. Effectors as tools in disease resistance breeding against biotrophic, hemibiotrophic, and necrotrophic plant pathogens. Molecular Plant-Microbe Interactions. 2014; 27 (3):196-206. DOI: 10.1094/MPMI-10-13-0313-IA - 44.
Mapuranga J, Zhang N, Zhang L, Chang J, Yang W. Infection strategies and pathogenicity of biotrophic plant fungal pathogens. Frontiers in Microbiology. 2022; 13 :799396. DOI: 10.3389/fmicb.2022.799396 - 45.
Salcedo-Sarmiento S, Aucique-Pérez CE, Silveira PR, Colmán AA, Silva AL, Mansur PSC, et al. Elucidating the interactions between the rust Hemileia vastatrix and aCalonectria mycoparasite and the coffee plant. Iscience. 2021;24 (4):102352 - 46.
Chen H, He S, Zhang S, Li W, Liu S. The Necrotroph Botrytis cinerea BcSpd1 plays a key role in modulating both fungal pathogenic factors and plant disease development. Frontiers in Plant Science. 2022;13 :820767. DOI: 10.3389/fpls.2022.820767 - 47.
Belisário R, Aucique-Pérez CE, Abreu LM, Salcedo SS, de Oliveira WM, Furtado GQ. Infection by Neopestalotiopsis spp. occurs on unwounded eucalyptus leaves and is favoured by long periods of leaf wetness. Plant Pathology. 2020;69 (2):194-204. DOI: 10.1111/ppa.13132 - 48.
Cho Y. How the necrotrophic fungus Alternaria brassicicola kills plant cells remains an enigma. Eukaryotic Cell. 2015;14 (4):335-344. DOI: 10.1128/ec.00226-14 - 49.
Glazebrook J. Contrasting mechanisms of defense against biotrophic and necrotrophic pathogens. Annual Review of Phytopathology. 2005; 43 :205-227. DOI: 0.1146/annurev.phyto.43.040204.135923 - 50.
Koeck M, Hardham AR, Dodds PN. The role of effectors of biotrophic and hemibiotrophic fungi in infection. Cell Microbiology. 2011; 13 (12):1849-1857. DOI: 10.1111/j.1462-5822.2011.01665.x - 51.
Cruz MFA, Rios JA, Araujo L, Ávila RF. Infection process of Pyricularia oryzae on the leaves of wheat seedlings. Tropical Plant Pathology. 2016;41 :123-127. DOI: 10.1007/s40858-016-0068-6 - 52.
Aucique-Pérez CE, de Menezes Silva PE, Moreira WR, DaMatta FM, Rodrigues FÁ. Photosynthesis impairments and excitation energy dissipation on wheat plants supplied with silicon and infected with Pyricularia oryza e. Plant Physiology and Biochemistry. 2017;121 :196-205. DOI: 10.1016/j.plaphy.2017.10.023 - 53.
Mittler R, Vanderauwera S, Suzuki N, Miller G, Tognetti V, Vandepoele K, et al. ROS signaling: The new wave? Trends in Plant Science. 2011; 16 :300-309. DOI: 10.1016/j.tplants.2011.03.007 - 54.
Bispo WMS, Araujo L, Ávila RT, DaMatta FM, Rodrigues FÁ. Mango resistance against Ceratocystis fimbriata is impaired by local starch mobilization. Tropical Plant Pathology. 2016;41 :225-236. DOI: 10.1007/s40858-016-0092-6 - 55.
Parker D, Beckmann M, Zubair H, Enot DP, Caracuel-Rios Z, Overy DP, et al. Metabolomic analysis reveals a common pattern of metabolic re-programming during invasion of three host plant species by Magnaporthe grisea . The Plant Journal. 2009;59 (5):723-737. DOI: 10.1111/j.1365-313X.2009.03912.x - 56.
Koch KE. Carbohydrate-modulated gene expression in plants. Annual Review of Plant Physiology and Plant Molecular Biology. 1996; 47 (1):509-540. DOI: 10.1146/annurev.arplant.47.1.509 - 57.
Essmann J, Schmitz-Thom I, Schön H, Sonnewald S, Weis E, Scharte J. RNA interference-mediated repression of cell wall invertase impairs defense in source leaves of tobacco. Plant Physiology. 2008; 147 (3):1288-1299. DOI: 10.1104/pp.108.121418 - 58.
Ayres PG. Responses of stomata to pathogenic microorganisms. In: Stomatal Physiology. Cambridge, UK: Cambridge University Press; 1981. pp. 205-211 - 59.
Berdugo CA, Zito R, Paulus S, Mahlein A. Fusion of sensor data for the detection and differentiation of plant diseases in cucumber. Plant Pathology. 2014; 63 (6):1344-1356. DOI: 10.1111/ppa.12219 - 60.
Bermudez-Cardona MB, Filho JAW, Rodrigues FA. Leaf gas exchange and chlorophyll a fluorescence in maize leaves infected with Stenocarpella macrospora . Phytopathology. 2015;105 (1):26-34. DOI: 10.1094/PHYTO-04-14-0096-R - 61.
Alves AA, da Guimarães LM d S, Chaves AR d M, DaMatta FM, Alfenas AC. Leaf gas exchange and chlorophyll a fluorescence of Eucalyptus urophylla in response toPuccinia Psidii infection. Acta Physiologiae Plantarum. 2011;33 :1831-1839. DOI: 10.1007/s11738-011-0722-z - 62.
Einhardt AM, Ferreira S, Souza GMF, Mochko ACR, Rodrigues FA. Cellular oxidative damage and impairment on the photosynthetic apparatus caused by Asian soybean rust on soybeans are alleviated by nickel. Acta Physiologiae Plantarum. 2020; 42 :1-13. DOI: 10.1007/s11738-020-03108-x - 63.
Muller P, Li X-PP, Niyogi KK, Müller P, Li X-PP, Niyogi KK. Non-photochemical quenching. A response to excess light energy. Plant Physiology. 2001; 125 (4):1558-1566. DOI: 10.1104/pp.125.4.1558 - 64.
Baker NR, Rosenqvist E. Applications of chlorophyll fluorescence can improve crop production strategies: An examination of future possibilities. Journal of Experimental Botany. 2004; 55 (403):1607-1621. DOI: 10.1093/jxb/erh196 - 65.
Honorato J, Zambolim L, Duarte HSS, Aucique-Pérez CE, Rodrigues FÁ. Effects of epoxiconozale and pyraclostrobin fungicides in the infection process of Hemileia vastatrix on coffee leaves as determined by chlorophyll a fluorescence imaging. Journal of Phytopathology. 2015;163 (11-12):968-977. DOI: 10.1111/jph.12399 - 66.
Spoustová P, Synková H, Valcke R, Čeřovská N. Chlorophyll a fluorescence as a tool for a study of the potato virus Y effects on photosynthesis of nontransgenic and transgenic P Ssu-Ipt tobacco. Photosynthetica. 2013; 51 (2):191-201. DOI: 10.1007/s11099-013-0023-4 - 67.
Debona D, Rios JA, Nascimento KJT, Silva LC, Rodrigues FA. Influence of magnesium on physiological responses of wheat infected by Pyricularia oryzae . Plant Pathology. 2016;65 (1):114-123. DOI: 10.1111/ppa.12390 - 68.
Silva BN, Oliveira LM, Mochko ACR, Chaves JAA, Aucique-Pérez CE, Rodrigues FA. Physiological and biochemical changes in wheat plants infected by Pyricularia Oryzae caused by thermal oscillations. Physiological and Molecular Plant Pathology. 2021;115 :101646. DOI: 10.1016/j.pmpp.2021.101646 - 69.
de Torres ZM, Littlejohn G, Jayaraman S, Studholme D, Bailey T, Lawson T, et al. Chloroplasts play a central role in plant defence and are targeted by pathogen effectors. Nature Plants. 2015; 1 (6):1-10. DOI: 10.1111/pce.12924 - 70.
Oliveira TB, Aucique-Pérez CE, Einhardt AM, Rodrigues FÁ. Wheat susceptibility to blast is enhanced by a photosynthetic inhibitor. Journal of Phytopathology. 2021; 169 (10):630-639. DOI: 10.1111/jph.13034 - 71.
Ajigboye OO, Murchie E, Ray RV. Foliar application of isopyrazam and epoxiconazole improves photosystem II efficiency, biomass and yield in winter wheat. Pesticide Biochemistry and Physiology. 2014; 114 :52-60. DOI: 10.1016/j.pestbp.2014.07.003 - 72.
Rousseau C, Belin E, Bove E, Rousseau D, Fabre F, Berruyer R, et al. High throughput quantitative phenotyping of plant resistance using chlorophyll fluorescence image analysis. Plant Methods. 2013; 9 (1):17. DOI: 10.1186/1746-4811-9-17 - 73.
Bürling K, Hunsche M, Noga G. Quantum yield of non-regulated energy dissipation in PSII (Y (NO)) for early detection of leaf rust ( Puccinia triticina ) infection in susceptible and resistant wheat (Triticum Aestivum L.) cultivars. Precision Agriculture. 2010;11 (6):703-716. DOI: 10.1007/s11119-010-9194-1 - 74.
Kuckenberg J, Tartachnyk I, Schmitz-Eiberger M, Noga G. Early detection of leaf rust and powdery mildew infections on wheat leaves by PAM fluorescence imaging. Precision Agriculture ‘07. 2007; 8 :515-521. DOI: 10.3920/978-90-8686-603-8 - 75.
Kim JH, Bhandari SR, Chae SY, Cho MC, Lee JG. Application of maximum quantum yield, a parameter of chlorophyll fluorescence, for early determination of bacterial wilt in tomato seedlings. Horticulture, Environment, and Biotechnology. 2019; 60 :821-829. DOI: 10.1007/s13580-019-00182-0 - 76.
Grishina A, Sherstneva O, Grinberg M, Zdobnova T, Ageyeva M, Khlopkov A, et al. Pre-symptomatic detection of viral infection in tobacco leaves using pam fluorometry. Plants. 2021; 10 (12):2782. DOI: 10.3390/plants10122782 - 77.
Moustaka J, Moustakas M. Early-stage detection of biotic and abiotic stress on plants by chlorophyll fluorescence imaging analysis. Biosensors. 2023; 13 (8):796. DOI: 10.3390/bios13080796 - 78.
Wang L, Poque S, Valkonen JPT. Phenotyping viral infection in sweetpotato using a high-throughput chlorophyll fluorescence and thermal imaging platform. Plant Methods. 2019; 15 :1-14. DOI: 10.1186/s13007-019-0501-1 - 79.
Aucique-Perez CE, Daza ES, Ávila-Diazgranados RA, Romero HM. Chlorophyll a fluorescence and leaf temperature are early indicators of oil palm diseases. Scientia Agricola. 2019; 77 :e20180106. DOI: 10.1590/1678-992X-2018-0106 - 80.
Mahlein AK, Alisaac E, Al Masri A, Behmann J, Dehne HW, Oerke EC. Comparison and combination of thermal, fluorescence, and hyperspectral imaging for monitoring fusarium head blight of wheat on spikelet scale. Sensors. 2019; 19 (10):2281. DOI: 10.3390/s19102281 - 81.
Sun Y, Liu T, Wang X, Hu Y. Chlorophyll fluorescence imaging combined with active oxygen metabolism for classification of similar diseases in cucumber plants. Agronomy. 2023; 13 (3):700. DOI: 10.3390/agronomy13030700 - 82.
He C, Li X, Liu Y, Yang B, Wu Z, Tan S, et al. Combining multicolor fluorescence imaging with multispectral reflectance imaging for rapid citrus huanglongbing detection based on lightweight convolutional neural network using a handheld device. Computers and Electronics in Agriculture. 2022; 194 :106808. DOI: 10.1016/j.compag.2022.106808 - 83.
Sapoukhina N, Boureau T, Rousseau D. Plant disease symptom segmentation in chlorophyll fluorescence imaging with a synthetic dataset. Frontiers in Plant Science. 2022; 13 :969205. DOI: 10.3389/fpls.2022.969205 - 84.
Martínez-Ferri E, Zumaquero A, Ariza MT, Barceló A, Pliego C. Nondestructive detection of white root rot disease in avocado rootstocks by leaf chlorophyll fluorescence. Plant Disease. 2016; 100 (1):49-58. DOI: 10.1094/PDIS-01-15-0062-RE - 85.
Liu F, Zhang M, Hu J, Pan M, Shen L, Ye J, et al. Early diagnosis of pine wilt disease in Pinus thunbergii based on chlorophyll fluorescence parameters. Forests. 2023;14 (1):154. DOI: 10.3390/f14010154 - 86.
Baghbani F, Lotfi R, Moharramnejad S, Bandehagh A, Roostaei M, Rastogi A, et al. Impact of fusarium verticillioides on chlorophyll fluorescence parameters of two maize lines. European Journal of Plant Pathology. 2019;154 :337-346. DOI: 10.1007/s10658-018-01659-x - 87.
Weng H, Liu Y, Captoline I, Li X, Ye D, Wu R. Citrus huanglongbing detection based on polyphasic chlorophyll a fluorescence coupled with machine learning and model transfer in two citrus cultivars. Computers and Electronics in Agriculture. 2021; 187 :106289. DOI: 10.1016/j.compag.2021.106289 - 88.
Petruccelli V, Brasili E, Varone L, Valletta A, Pasqua G. Antifungal activity of dimethyl sulfoxide against Botrytis cinerea and phytotoxicity on tomato and lettuce plants. Plant Biosystems - An International Journal Dealing with All Aspects of Plant Biology. 2020;154 (4):455-462. DOI: 10.1080/11263504.2020.1779846 - 89.
Suárez JC, Vanegas JI, Contreras AT, Anzola JA, Urban MO, Beebe SE, et al. Chlorophyll fluorescence imaging as a tool for evaluating disease resistance of common bean lines in the western amazon region of Colombia. Plants. 2022; 11 (10):1371. DOI: 10.3390/plants11101371 - 90.
Warzecha T, Skrzypek E, Adamski T, Surma M, Kaczmarek Z, Sutkowska A. Chlorophyll a fluorescence parameters of hulled and hull-less barley ( Hordeum vulgare L.) DH lines inoculated withFusarium culmorum . The Plant Pathology Journal. 2019;35 (2):112. DOI: 10.5423/PPJ.OA.07.2018.0124